What data tells us about the trends shaping healthtech
In brief
Matthieu Vallin takes a granular look at three years’ of available funding data to ask how the early-stage healthtech landscape is changing. The data shows that priorities are changing – the clinical element of AI is ever more important and scrutinised, consumer health platform are becoming more medicalised into virtual clinics, the lines between AI / automation / practice management software are blurring, and generative & large AI models are set to transform the entire care continuum – from patient screening to payer reimbursements.
He also asks what this data can tell us about the future. Investment areas likely to win big in the years to come include clinical-grade AI, workforce management platforms, hybrid care models, remote monitoring solutions, and regulatory & compliance. Other areas have slipped, with consumer health, insurance/finance and telehealth attracting less funding than previously.
Contents
2.1 From medical device certification to marketing compliance – regulatory SaaS will have a big year
2.2 LLM stack: where does value lie?
2.3 Remote monitoring adoption with better respiratory, cardiac and neuro biomarkers and care
2.4 Privacy preserving infrastructure to enable data collaboration
2.5 Invasive vs. non-invasive sensors: the dance continues
2.7 Consolidation of clinical AI, automation and practice management software
2.8 Healthcare staff crunch, hybrid healthcare and practicing at the top of licenses
Digital health platforms & SaaS funding: looking back and looking forward
Building up a clear picture of the shape things might take is always a challenge, especially given the tempestuous economic weather of recent years. But we wanted to form a measured view of future funding hotspots in 2024 – and beyond. We’ve looked back to review available funding data from the last three years for a clear understanding of the trends shaping digital health, to discover which are growing – and which are contracting.
The years since 2020 have been a funding rollercoaster, but as we explored in our 2023 report, The Resilience of Early-stage European Healthtech, the early stages have proved resilient. Between 2020 and 2023, we’ve seen consistency in Seed to Series A investment. It’s not all good news: growth rounds and mega-rounds (of $100m plus) are scarce. Exits and IPOs also remain low.
Still, last year founders and entrepreneurs displayed incredible resilience and resourcefulness, moving at speed to adapt their funding strategies and runways to meet the demands of the funding environment. Despite tougher macro conditions, we’ve seen top-performing countries raising outlier rounds. These firms continue to enjoy attention from investors, choosing between competing term sheets and taking their pick, based on the attractiveness of the deal or value alignment.
In this deep dive, I want to leverage funding data to ask how the investment landscape is changing. Which segments are emerging as winners – and where will we at Octopus Ventures be aiming to invest in the months and years to come.
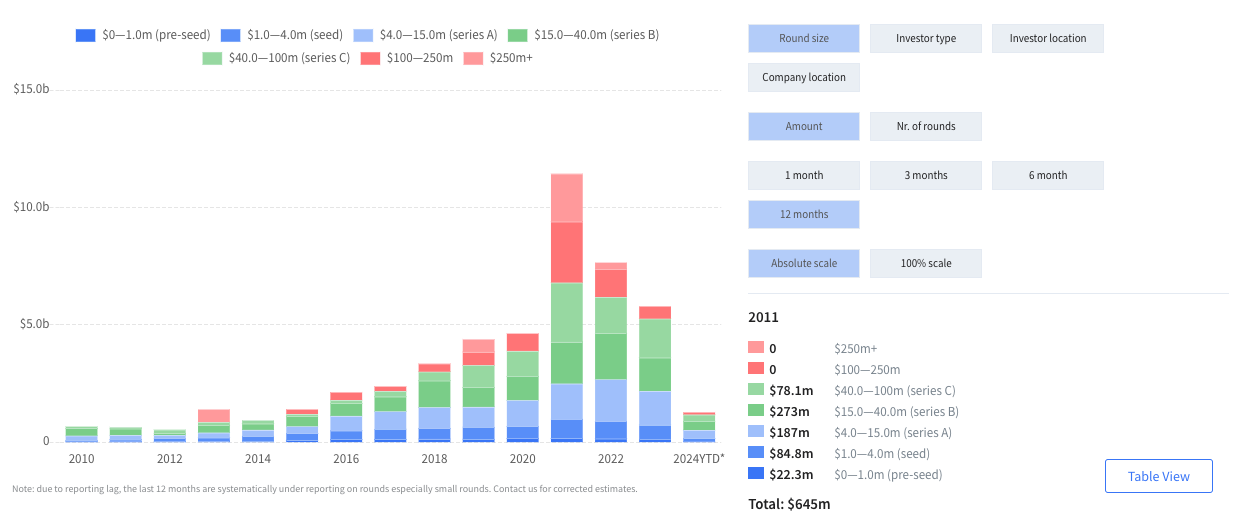
Looking back:
To build up a clear understanding of how healthtech investors are allocating their capital, we classified digital health funding rounds that achieved a minimum of $1m total funding from 2020 to 2023 into twenty categories. These are: clinical AI, clinical trials, consumer health, data infrastructure, digital therapeutics, elderly care, employer-led health, health logistics, hybrid healthcare, insurance/fintech, life sciences tools, practice management platforms, regulatory & compliance, medical device diagnostics, remote diagnostics and monitoring, surgical guidance and robotics, telehealth, virtual care provider and workforce management and education.
The data shows a shift in investment focus. Direct comparison of the top investment categories of 2021 and 2023 reveals that certain focus areas have shifted up the list of priories. The fastest moving categories were clinical AI, medical device diagnostics, health logistics and workforce management and education.
At the same time, others have moved down. These include consumer health, telehealth and insurance/finance. So what can we infer about the priorities of founders and investors?
- Clinical-grade AI & point solutions: Even at early stages, there is an increasing focus on companies that have demonstrable clinical evidence. This can take the form of peer-reviewed papers for their technology, clinical studies/trials, and/or a defensible IP portfolio and strategy. This observation is echoed in the US. Galen Growth found that last year there was a shift in focus in proof points, with clinical strength being a priority data point. I’m biased here, but Octopus Ventures portfolio companies Ibex Medical Analytics (imaging AI in pathology) and Ufonia (clinical conversational AI) are supporting this claim. Since their inception, both have invested in becoming the clinical AI leaders in their respective spaces. Now, they’re signing major customers in public and private health. Elsewhere, companies such as BrightHeart and Fraiya’s point solutions are focussing on pre-natal ultrasound AI, which could underpin a step-change in early-life diagnostics. Last year, best-in-class clinical AI point solutions attracted both customers and funding. We’re anticipating more of the same in 2024.
- Consumer health evolving into virtual care providers: Factors including decreased consumer spending, high inflation and low valuations have seen consumer health take a big hit. But category leaders are well-funded and many are prescribing drugs themselves, becoming, in turn, clinical-grade virtual care providers. Oura searches for new sleep biomarkers, while Manual prescribes GLP-1s efficiently through a holistic weight loss programme. At the same time, the technical gap between wearable data and other healthcare infrastructure is shrinking, due to promising companies like Thryve and Terra whose innovations are bridging the gap. Prevention is a consumer play, so these well-funded companies need to be engaging with patients at the top of the diagnostic funnel.
- Surgical Guidance & Robotics: It’s hard to believe that operating rooms are really coming to look how they do (see Fig. 1). Thanks to the lofty backing of businesses like CMR Surgical, Distalmotion, Proximie and Moon Surgical (amongst others) the progress on the surgical robotics & decision-support front has been phenomenal. It was also consistently backed from 2021 through to 2023, making it one of the leading European categories in consecutive years. Deep tech companies are leveraging new technologies to offer enhanced surgical assistance. Hypervision Surgical are developing hyperspectral imaging to help surgeons distinguish between tissue types in near real time. Others, like Cydar Medical, provide AI-assisted 3-D renderings of vasculature to assist surgeons in surgical preparation and decision support. Virtual reality (VR) and augmented reality (AR) have also found a product-market fit in surgical education, preparation and guidance. Over the last three years, the sector has maintained steady, elevated funding levels, which, as it becomes a more established category, see it suited to private equity or growth funds. We’re not expecting any further mega-rounds in the space in 2024, but we’re happy to see the tremendous capital operating rooms have received in the last three years.
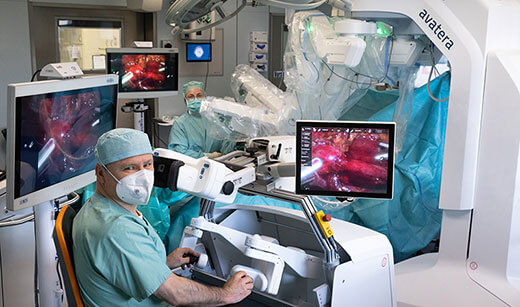
- Health data infrastructure is close to taking off, but faces strong headwinds: The simple fact is that the technology is moving faster than customers’ adoption speed. Pioneering companies like the French Arkhn or Dutch Founda Health, Spanish Iomed or the UK’s Cogstack have the infrastructure solutions that providers, hospital networks and pharma desperately need. These solutions improve data access, cleansing, standardisation, sharing and analytics across health systems’ organisation and stacks, enabling secondary uses cases, from informing clinical studies to improving existing clinical services. Companies (including Iomed) are doubling down, and rightly so, offering support to providers through specific training sessions, teaching them to adopt data standardisation protocols and common data models, which serve as the basis for a range of exciting activities, such as pioneering observational studies. The companies innovating in the foundations and tooling of health data show huge promise, but are slowed by the gigantic scale of the digital transformation projects needed to migrate from a legacy data infrastructure, as well as typically slow-moving national programmes. Major players like Palantir, who won the bid for the NHS England Federated Data Platform project, in turn become an attractive channel partner for startups. Currently, health systems and infrastructure startups are living on different timelines. Six to 12 months is a workstream for one, versus a lifetime for the other. We admire the resilience of these companies – they haven’t (yet) been rewarded with the sharp commercial uptake they deserve for the value they create.
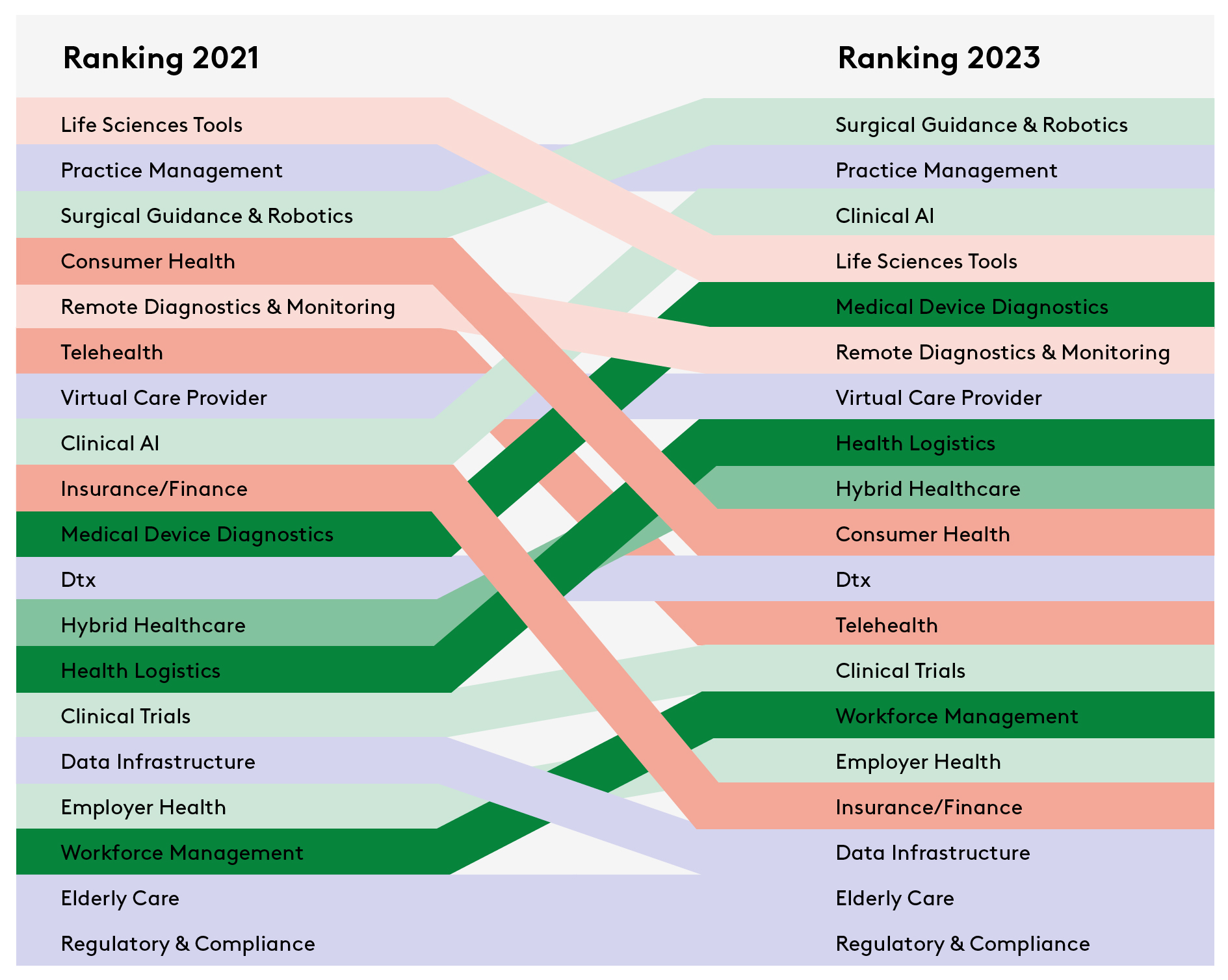
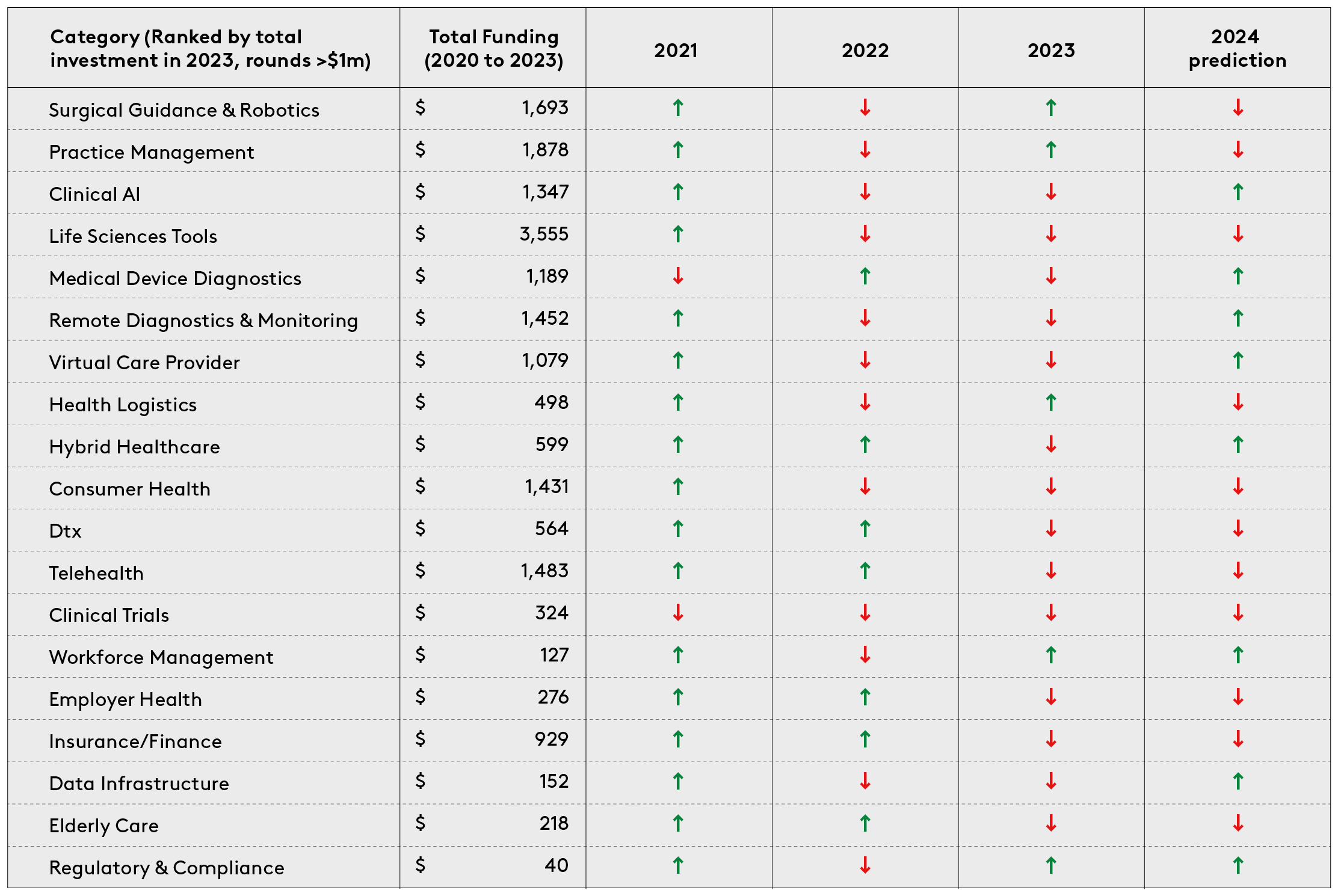
Looking forward – and what we’re looking for as a fund:
We’ve seen what the weighting of funding allocation does for investment segments and what it says about the priorities of investors, customers and founders alike. But what can we learn about the future?
Based on the shifting focus of the last three years, here’s how the next might shape up – and where Octopus Ventures fits into it.
1. From medical device certification to marketing compliance – regulatory SaaS will have a big year:
- Likely to be one of the highest growth categories in 2024 and 2025, with large Series A and Series B rounds raised by five to 10 companies in Europe. In 2020, the category was still in its infancy, with roughly $2m in annual funding. This grew 10x in 2022, reaching total funding of $20m. Early-stage companies are innovating across different segments within the regulatory & compliance space, at different stages of product development and product lifecycle. Flinn, for example, is focusing on life cycle requirements & post-market surveillance automation to improve the launch & monitoring of medical software. Scarlet have become the first Notified Body to specialise on medical software & AI regulation, after gaining the sign-off from the Medical Device Coordination Group (or MDCG – a group that essentially oversees the governance and performance of notified bodies in the EU). Scarlet’s certifications process should catalyse real innovation in clinical AI development & adoption, as they close the gap between the speed of product development and regulation – all of this to the benefit of patients. Other healthcare regtech companies to keep an eye on are: Onside.ai, Cleary.ai, Formly.ai, Worldover.io and RegMetrics – all at pre-seed & seed stage, and will likely raise bigger rounds this year, considering the problem statement is getting worse between regulators’ capacity vs workload.
- We’re seeing major innovation across other heavily regulated pathways, such as medical device & pharma marketing. EU and local regulations are strict about what pharma companies can and can’t say. Legislation is constantly evolving and offers little margin for error, with tough repercussions for the companies that get it wrong. French front-runner SaaS company, Ubaq (a merger of Qairn and Clardian), automates the medical, legal and regulatory processes of healthcare marketing by enabling different parts of healthcare companies to work together, right the way from content creation through regulatory submission, massively improving the efficiency and quality of pharma & medtech marketing. Earlier-stage companies like YC-backed Sei (also focused on AI-led marketing compliance) take a different approach, providing a co-pilot built on fine-tuned open-source large language models (LLMs), guiding their users and clients through the process.
2. LLM stack: where does value lie?
When it comes to the extraordinary advances in AI, and the seemingly limitless opportunities created by LLMs, the key question to ask is, where does value truly lie? From foundation models to the infrastructure layer, AI system design, enablement layer to direct applications, how and where will we see LLMs being adopted at scale within the healthcare industry?
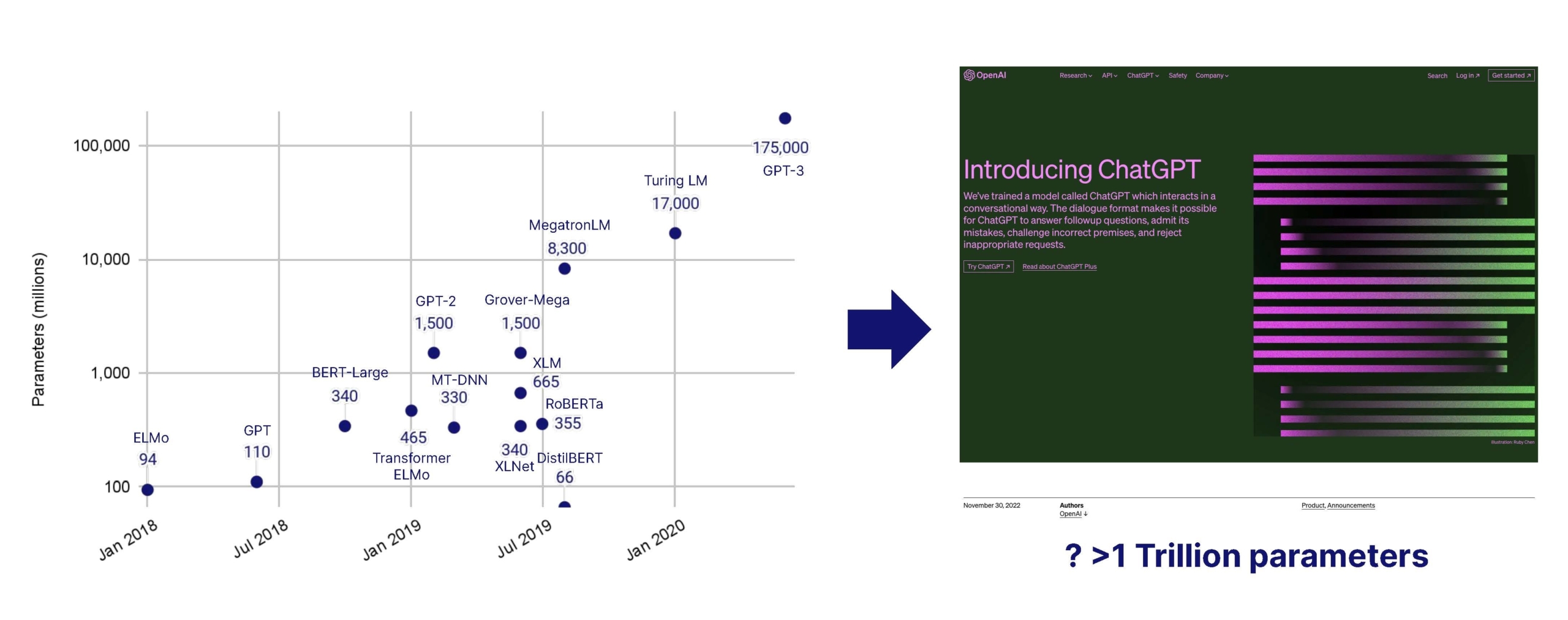
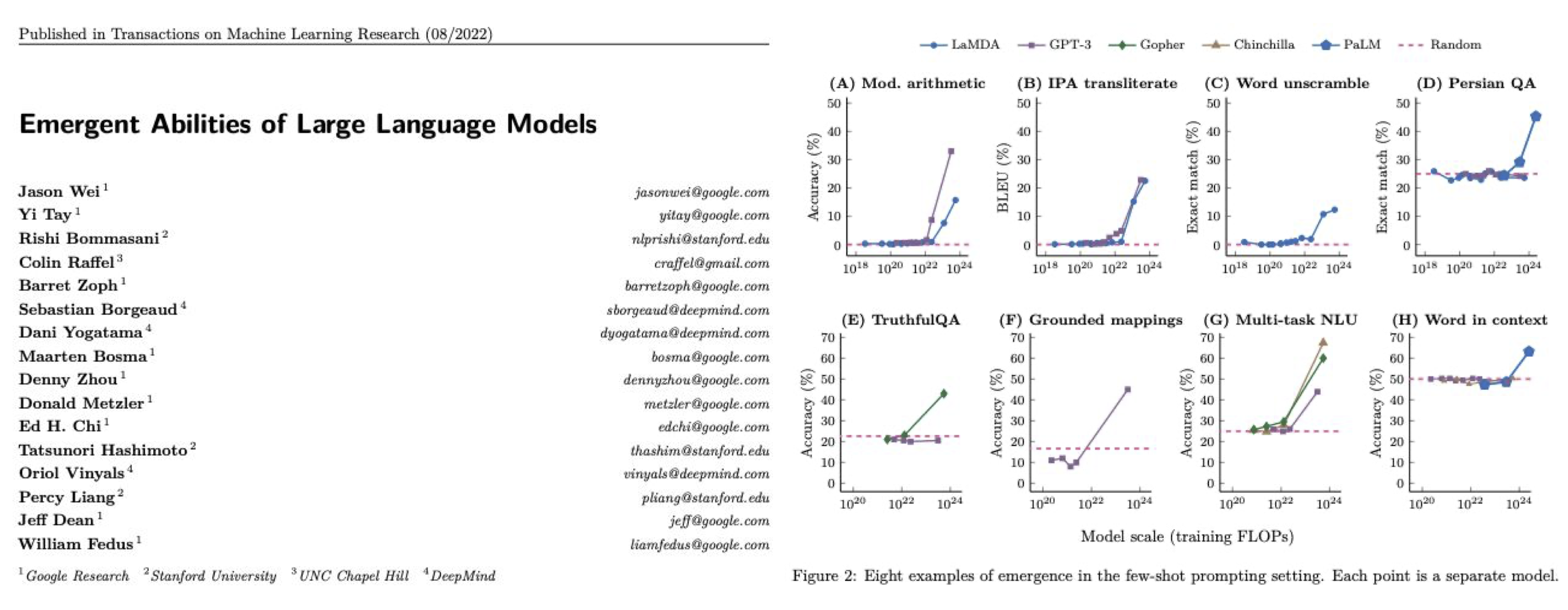
- Natural language processing, LLMs, and LMMs. With so much of healthcare data held in legacy systems in a text-based format, the industry lends itself well to natural language processing, through large AI (including large language models) which are booming in parameters, usability and accuracy. With imaging playing a critical role across most specialities, large multi-modal AI models can be a game changer to collate data across the patient journey to make better diagnostic and treatment decisions. Use of older large AI models has been widespread for several years, but the recent surge in LLM performance, usability and generative capabilities represents a major innovative leap. The application layer has grown massively, with the arrival of countless co-pilots for all parts of healthcare, finance and administration. We’re excited to see them crack distribution across health systems, which is usually on the slower end of the technology adoption curve. Top-down competition is fierce in the space, with players like Microsoft owned Nuance, which can roll-out ChatGPT co-pilots across its very large install base.
- Emerging applications. The PromedAI team has worked with other entrepreneurs and researchers to put together this great overview of research into medical LLM training, fine-tuning, applications and generative tasks. It shows performance varying across clinical applications by model and modality (text, voice, image, etc). Currently, the most common and most researched applications are: medical diagnosis, formatting and ICD-coding, clinical report generation, medical education, medical robotics, medical language translation and mental health support. Here are a few of the pioneering companies cutting across these use cases that we’re excited by: Phare Health, Co-Helm, Sohar Health, Tortus and Promed. There are, of course, many more. To stay up-to-speed with rapidly moving developments in the space, I recommend signing up to the Neural Medwork, a recently launched newsletter dedicated to ‘decoding AI for healthcare professionals’.
- Foundation models. The foundation models behind these LLMs are inherently valuable but they tend to be owned by, adjacent to or associated with big tech companies. Outlying pioneers like Hippocratic AI, Mistral and Haiper.ai (an OV-backed business) have received mostly private funding from VCs and are already on to mega-round territory – less relevant for early-stage investors. Industry giant OpenAI has already reached the $2bn revenue mark, which is nothing short of a technology breakthrough. We’re keeping an eye on emerging types of models, transformers and new model architectures, with different underlying machine learning methods, training data moats and compute efficiency. For example, a recent exciting seed-stage investment is BioOptimus led by Sofinnova ($35m seed round announced in February 2024), which has a unique access to mutli-modal biological and patient data through Owkin’s federated network, alongside other data partnerships.
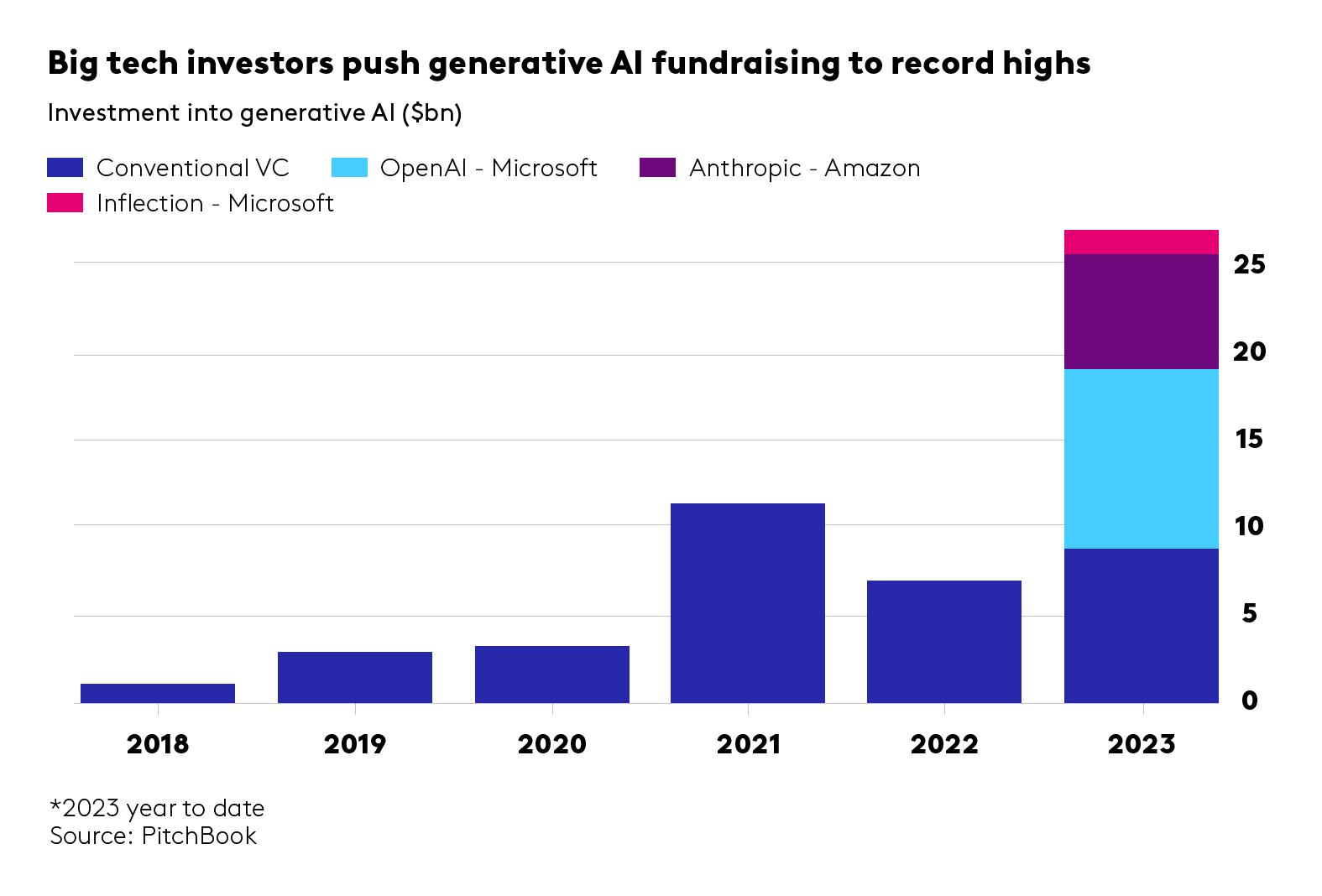
- Fine tuning and RAG on proprietary data to achieve clinical value and adoption. Most healthcare companies sit on a wealth of valuable proprietary data, gathered across studies and trials, from patients and clinicians. Leveraging it for training open-source models allows businesses to fine-tune them, developing healthcare applications with clinical value and low error rates, safe and suitable for clinical practice. Other prompt-engineering techniques, like Retrieval Augmented Generation (RAG), improve the efficiency and accuracy of an NLP or LLM task by retrieving data or documents from outside the LLM to provide it with augmented context and information and so improve the task output accuracy. A good example is offered by Octopus Ventures backed company, Ufonia. They sit on a very wide set of ophthalmology patient data and use it to streamline patient services with their automated conversational modules, built to replace routine pre- and post-op appointments in public and private health. The company’s clinical trials have demonstrated acceptability to patients and clinicians. We’re anticipating use of proprietary data and third-party data with open-source models, paired with innovative prompt engineering techniques, to increase the clinical relevance of LLMs. The challenge is how to scale these systems cost-efficiently while optimising for safety and clinical KPIs (see below). Writing candidly, the extension of LLMs with large multi-modal models (LMMs), using new inputs such as image/visual analysis is fascinating. With the right training and clinical/regulatory validation, tools like GPT4-V (vision) could combine image data with clinical context to inform healthcare screening, diagnostics and treatment plans. There’s still a long way to go, but error rates across specialties will only reduce over time with the right training.
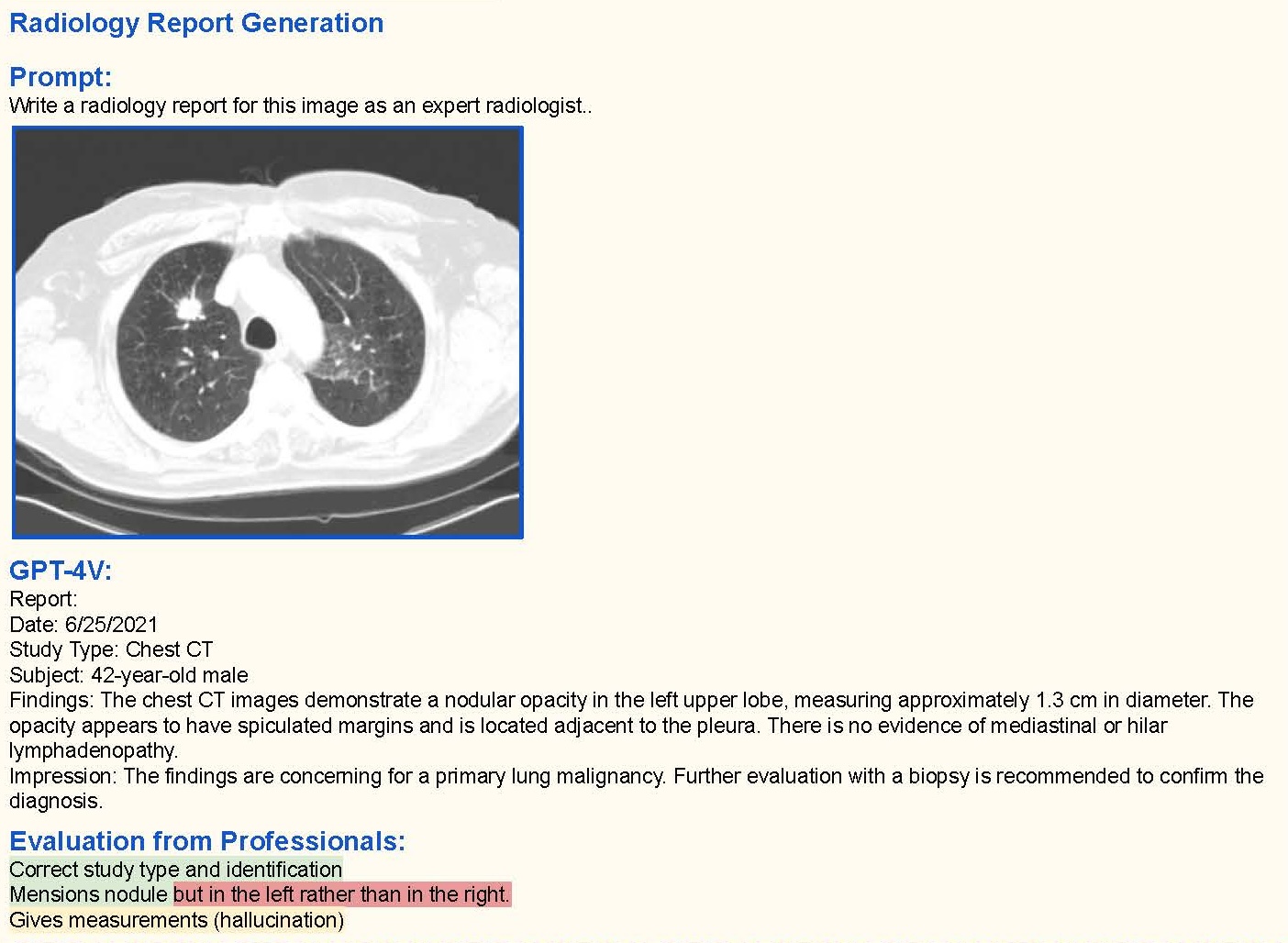
- Enablement layer, data privacy and security. Security remains a concern. There’s still much uncertainty around just how companies’ existing data and data infrastructure can interact with these large AI models. In fact, 74% of IT decision makers are concerned with the security risks of GPT-4. For example, an LLM might inadvertently reveal confidential company information in responses (see Fig. 6).
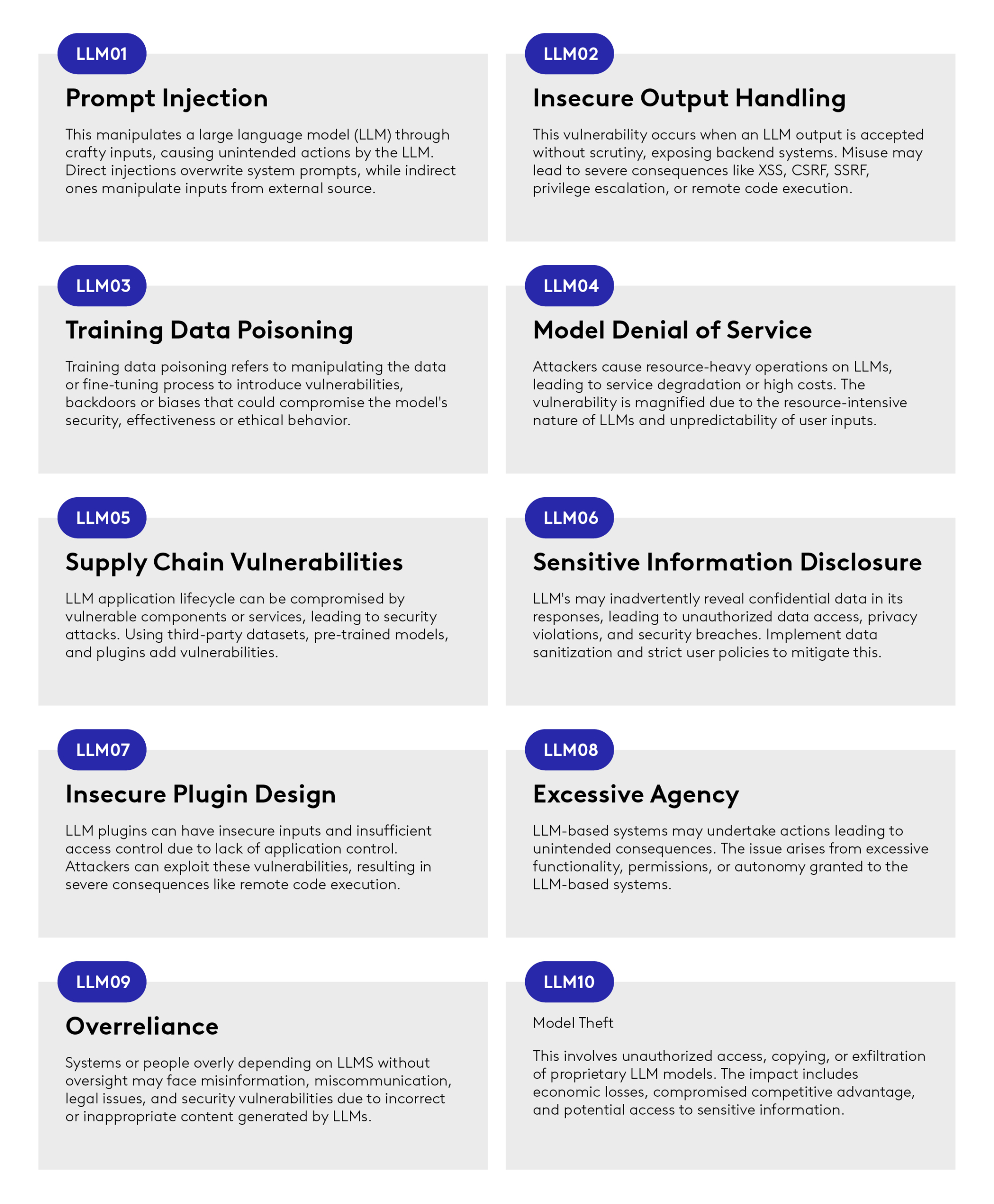
- Cross-department collaboration. New technologies require a multitude of departments to work together, and if LLMs are going to find widespread adoption, everyone from data science teams and data security to legal and regulatory will need to collaborate on finding answers to questions like: what data can a GP input into an LLM? What format should it be? Which LLM should they use, and which should they avoid? How do they keep an audit trail of decisions made by AI? How does an organisation provide the guardrails to manage model hallucination or erroneous outputs to its clients? These can be answered with the right tooling and the right infrastructure, to join the dots between the healthcare infrastructure that’s already in place and the large AI ecosystem. Companies like Puppeteer.ai are working on this by prioritising compliance, patient safety and deep integration – both front-end (with existing patient communication tools) and back-end (into electronic health records (EHRs)). The company provides built-in modules and integrations that enable organisations to interact with multiple LLMs to improve patient interactions and clinical care, while providing the necessary data security measures like HIPAA compliance. We expect these enabler companies to be the glue between the different tech stacks, which today are loosely interacting in sensitive clinical environments.
- Compound AI system design & LLMOps: As mentioned recently in a post from the Berkeley Artificial Intelligence Platform (BAIR), ‘State-of-the-art AI results are increasingly obtained by compound systems with multiple components, not just monolithic models.’ A typical AI task requires different components to complete. As a simple example, to augment an LLM’s capabilities, prompt engineering techniques such as RAG (discussed above) involve a retrieval model on top of the LLM, which in turn creates a system (see Fig. 7). This architecture opens the door for various system designs, which influence the cost, resources, efficiency, accuracy and scaling potential of an AI task. For example, how often should the system call the LLM? Should the retriever call upon other LLMs? If so, with which frequency and sequencing? All these design choices have an impact on the efficiency and accuracy of the model. Making compute cost non-linear with model performance will be a key challenge over the next few years. We’re interested in healthcare-adjacent start-ups that provide the tooling for system and task design, to help healthcare organisation better optimise their AI tasks and correctly allocate the right resources and investment in their LLMOps infrastructure. For more information on AI infrastructure, check out www.AI-infra.fun
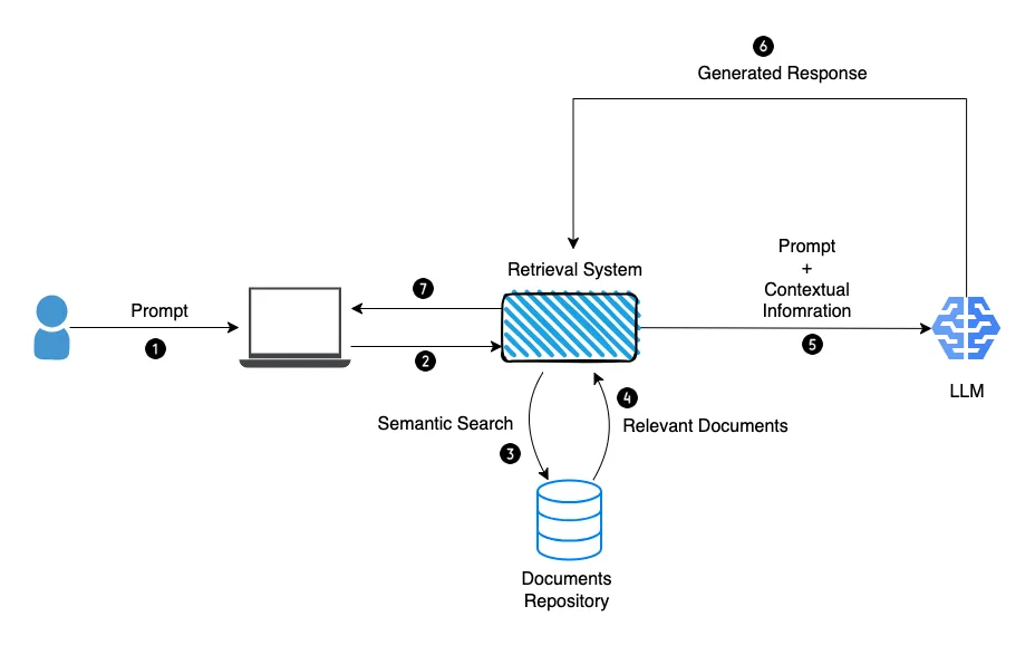
3. Remote monitoring adoption with better respiratory, cardiac and neuro biomarkers and care
In the UK today the remote monitoring market is fragmented across disease-specific solutions, at-home care and staffing platforms and practice management software. Companies like Doccla and Feebris are consolidating the value proposition and growing at pace within ICS’s and Trusts, providing at-home monitoring hardware, practice management software and care/support teams to ensure patients are taken care of.
Vital signs monitoring and other more traditional markers have been around for decades, but recent advances in clinical-grade at-home devices will broaden the potential of at-home care. NuvoAir, for example, have received 510(k) (premarket submission to the US Food and Drug Administration, or FDA) clearance to bring the accuracy and reliability of clinic-grade spirometry to patients at home. Similarly, Neu Health has developed digital mobility and cognitive markers for Parkinson’s and Dementia care, to develop predictive insights on symptoms including falls, gait freezing, postural instability and cognitive decline. Recently, the Samsung Galaxy Watch received FDA approval to diagnose sleep apnoea, a precursor to serious heart conditions and historically a challenge to diagnose outside clinical environments.
Video and image-based markers are also being developed to measure things like blood pressure. The challenge of measuring clinical-grade systolic and diastolic pressure from image and video alone remains, although the accuracy of the models is trending in the right direction (see: Binah.ai and Lifelight). PocDoc are innovating across at-home cardiovascular pathways by providing proprietary, five marker cholesterol lateral flow tests with an image-based AI tool to provide in-app results for the patient. This, in turn informs clinical teams of results and concerns.
We expect companies like Doccla and Feebris to become the go-to-market channel by which pioneering and regulated devices/biomarkers reach a wide patient base and truly enable remote care across disease areas.
4.Privacy preserving infrastructure to enable data collaboration
Without the right infrastructure, governance, security and incentives it’s going to be impossible to facilitate data sharing between providers, research institutions and pharma/medtech. The authors of this article in Nature put it perfectly when they wrote: ‘The lack of publicly available multi-centric and diverse datasets mainly stems from confidentiality and privacy concerns around sharing medical data’.
As discussed earlier, access to proprietary and third-party data will be critical for fine-tuning AI models and increasing the accuracy of diagnostics or treatments. This data, usually in different formats and standards, needs to not just ‘move’ between internal healthcare systems, it also needs to exist within an ecosystem of third parties – between a remote monitoring platform and a provider, for example.
Companies like Owkin and Iomed offer their clients a safe data ecosystem across hospitals sites, helping them acquire, structure, aggregate and analyse a wide breadth of patient, clinical and trial data. This can improve client use cases, be it clinical decision support, drug development, patient monitoring or performance analytics of their own services. Apheris (OV backed) is a federated learning platform allowing privacy-compliant training of ML models for pharma. It is an end-to-end platform that enables clients to conduct analysis using sensitive data, without compromising the privacy or security of the data subjects.
Differential privacy techniques also look promising and can be integrated into federated learning platforms. These aim to protect the privacy of individual data points in a dataset while still allowing for meaningful analysis and learning. This is achieved by adding precisely calibrated noise to the output of computations or queries on the dataset. As a technique, differential privacy looks to be particularly useful in histopathology and medical imaging. We’re keeping a close eye on the emerging AI compliance, security and governance stack in healthcare this year, and we’re keen to talk to companies in the space.

5. Invasive vs. non-invasive sensors : The dance continues.
We’re continuing to see exciting progress in the development of non-invasive sensors offering new ways to diagnose or treat disease. It is difficult to predict whether non-invasive will prevail over invasive, but even so pioneering solutions, built on groundbreaking research, are emerging.
In the invasive corner, we’re seeing developments in the fertility and Assisted Reproductive Technology space (ART). Impli has developed a subcutaneous, aptamer-based sensor that can detect hormonal levels in real-time. This will be particularly helpful alongside ART procedures such as IVF, which demand regular blood draws to tailor hormonal stimulation protocols. Hormonal levels vary widely throughout the day and night, so there is a strong unmet need for patients and clinicians to acquire better continuous data and, in turn, provide better outcomes such as increasing pregnancy rates or live birth rates.
In the non-invasive corner, researchers at Caltech are prototyping a sweat-based aptamer sensor which can also measure hormonal levels.
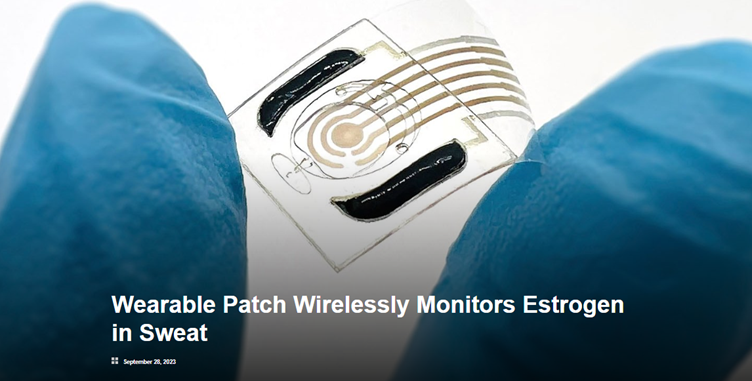
In the neuro space, companies like Nuuron and Cognito Therapeutics have developed non-invasive devices to reduce the speed and severity of cognitive decline for Alzheimer’s patients. Nuuron delivers a photonic stimulation through the retina, while Cognito provides auditory, visual, and vibration stimulation to affect the cognition/memory pathways. We’re excited to see further clinical trials of these technologies (alongside more approved drugs), to help improve the quality of life of people with these debilitating conditions.
There’s been major progress in the cardiac space, particularly on heart failure, where non-invasive bio-electronic medicine is set to have a big impact. UK-based company Parasym has gathered positive clinical data in treating heart failure. Their device improved cardiac mechanics and reduced inflammatory markers using non-invasive neuromodulation.
Unfortunately, there have also been some major setbacks, with clinical trials failing to demonstrate sufficient efficacy in the non-invasive treatment of auto-immune conditions, notably the treatment of rheumatoid arthritis using non-invasive vagus nerve stimulation. With the eternal trade-off set between patient safety, regulatory hurdles, clinical uptake and patient acceptability, the dance between invasive and non-invasive continues.
6. Digital therapeutics & virtual care platforms – buyers asking for more breadth and depth from vendors
Digital therapeutics (dtx) seems to be at a stand-still, with considerable consolidation happening in the space. We’re seeing large mental health platforms providing a combination of digital therapeutics and telehealth consultations. The distressed acquisition of Pear Therapeutics and the slow clinical uptake of DiGA-approved platforms in Germany have hit the dtx space hard in Europe and the US, but patient benefits remain, and digital-based programmes still carry serious potential. Buyers are being overwhelmed with point solutions in the mental health or musculoskeletal (MSK) space and demanding more from providers. That means a wider breadth of conditions covered, plus multiple touchpoints with patients (Digital therapeutic programmes, telehealth consultations, at-home monitoring and AI-led companions).
Still, we’re optimistic about the adoption of dtx. Interventions like cognitive behavioural therapy and acceptance therapy are well suited to the boost offered by LLMs capable of holding a conversation with users and providing them with adequate support. The distribution channels are in place (Spring Health, Big Health, Lyra Health) with self-insured employers and payers in the US. It falls to dtx providers to demonstrate strong patient uptake as well as positive clinical and health economic benefits.
In Europe, national health coverage of digital therapeutics is becoming a reality. Big Health’s Sleepio (Octopus Ventures backed) became the first ever digital therapeutic to receive guidance from the National Institute for Health and Care Excellence (NICE) in 2022. As patient and clinicians grow more used to clinical-grade digital programmes, we anticipate major uptake in 2024 and 2025.
7. Consolidation of clinical AI, automation and practice management software
Last year we saw large growth rounds for speciality specific practice management software, such as Ocuco in the Ophthalmology space (with a €60 million round). We also saw large growth rounds for the platforms providing a far wider set of practice management modules, including top-of-funnel patient ingestion, AI-augmented patient interactions, clinical workflow automation and medical billing and coding. Companies such as Corti can provide most of these solutions to their clients with a highly modular and effective practice management platform.
Similarly, Anima Health provides a very wide set of modules within its practice management platform, starting with total patient ingestion and running all the way through to the development of specific care plans, as well as patient communication. All of this without losing essential patient information, such as clinical context around symptoms.
Still, keeping patients from disappearing if they drop out of a platform (if they get referred to a secondary care service, for example) remains a challenge, which is why these highly modular and interoperable platforms need to move at speed and integrate throughout the care continuum, from early screening and diagnostics to patient discharge.
We expect these ‘total patient journey’ platforms to consolidate the market and acquire specific point-solutions at different ends of patient pathways, such as patient discharge solutions, asset tracking software within hospitals (wheelchairs, monitoring units, surgical equipment etc), epidemiology monitoring platforms and more.
8. Healthcare staff crunch, hybrid healthcare and practicing at the top of licenses
Private equity firms are investing heavily in the private healthcare providers that public health systems will have to turn to if they’re going to manage patient waiting lists and deliver the care people need. In the UK, clinical capacity is stretched across care pathways, while the country’s National Health Service (NHS) saw 40,365 NHS nurses leave active service in 2022 alone.
In this context, the part-time and full-time job markets need better underlying technology to match vacancies with professionals. Leading companies like Hublo and Patchwork Health have raised strong rounds (about $25m each in 2021 and 2022 respectively) to improve workforce management, staffing, analytics and payments across complex healthcare systems and siloes. We expect large growth rounds in this space in 2024 as staff shortages mount and clinical and administrative teams are stretched even further.
Another solution to this issue is to upskill healthcare professionals, offering training so that they can deliver a broader range of services. Octopus Ventures backed Tympa Health offers training to pharmacists and other staff, so that they can provide ear and hearing care services, including a digital otoscopy, ear cleansing through micro-suction and a hearing assessment. Without the Tympa training and medical device, these healthcare professionals could not be delivering this type of care, adding more strain to the NHS, where GPs are no longer trained to deliver these ear and hearing care services.
We want to keep investing in pioneering hardware/software plays that rewire existing patient pathways, with business model innovations at the core. We’ve seen similar promising startups in ophthalmology (Okko Health), dentistry/orthodontics (32Co) and gynaecology/endocrinology (Overture) where a combination of medical device innovation and new business models are establishing new routes to bring the right care to the right patient, short-circuiting or leap-frogging existing, inefficient pathways.
An even more daring approach is offered by the likes of Avi Medical, Jutro Medical and Patient21. These businesses are innovating on all fronts, from bricks and mortar to infrastructure, practice management software to screening/diagnostics, instead of trying to fix decades of tech debt and legacy systems, which don’t serve patients or staff well. A more traditionally PE investment opportunity has become attractive to venture capital funds, and we expect more mega round in 2024 and 2025 as these well-funded business pave a way to profitability.
Conclusion:
Close scrutiny of three years of investment data has given us a unique insight to the trends shaping healthtech today. As we’ve seen, emergent, step-change technologies carry incredible disruptive potential, with the power to reshape markets and capture capital almost overnight.
Looking ahead, we think each of these areas shows significant promise in opening fresh, innovative pathways with the potential to reshape patient care – and even remodel healthcare systems themselves.
At Octopus Ventures, we’re always open for innovation. We believe that healthtech genuinely has the power to change the world, and we’re fully committed to supporting the pioneers working to bring these new technologies and approaches into the market. If you’re working in any of the areas I’ve discussed and have a solution you think we need to know about, please reach out. You can learn about what our support looks like on our website. Pitch us here, or get in touch. You can reach me on [email protected]